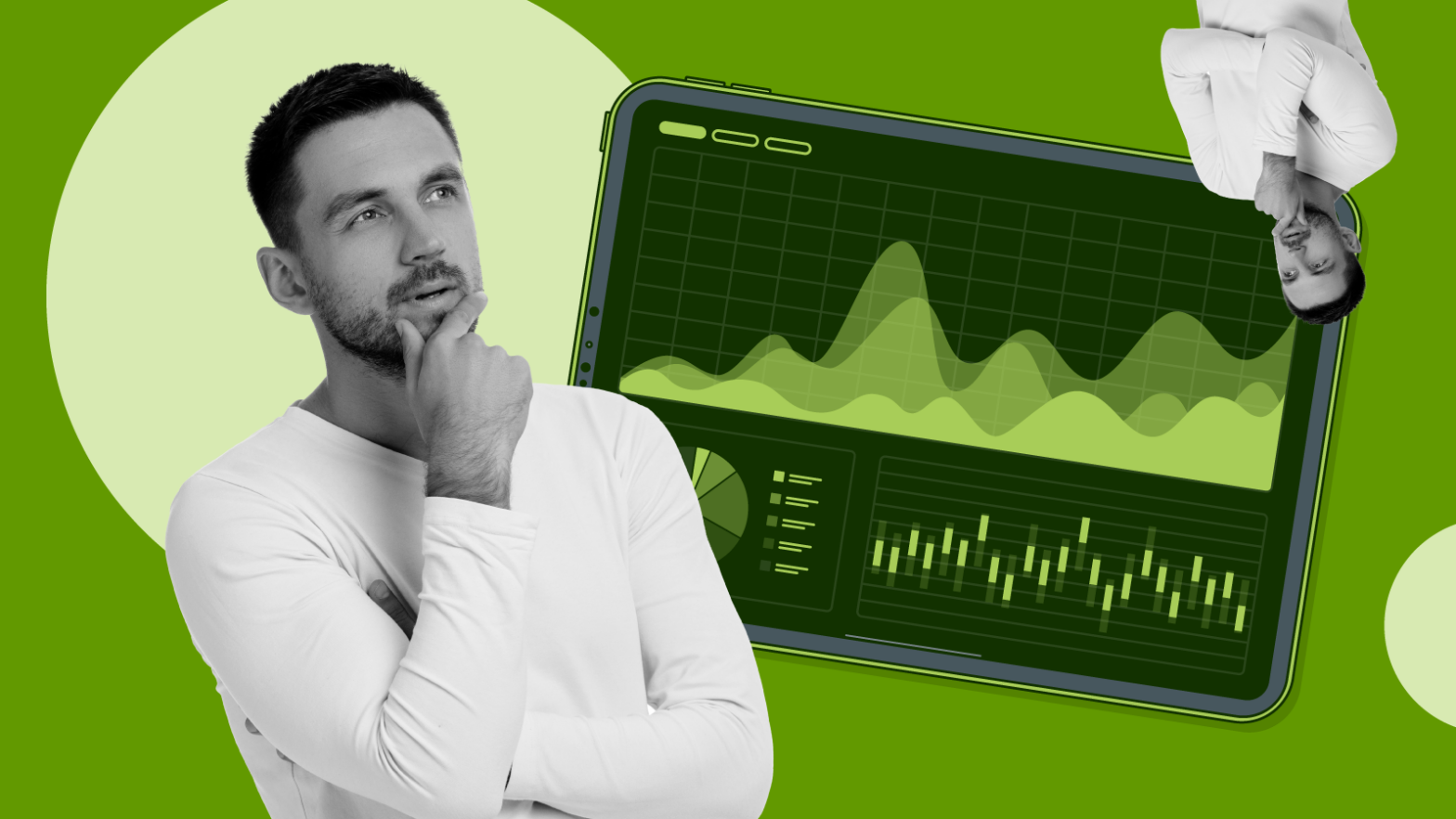
HR data analytics – Essential insights
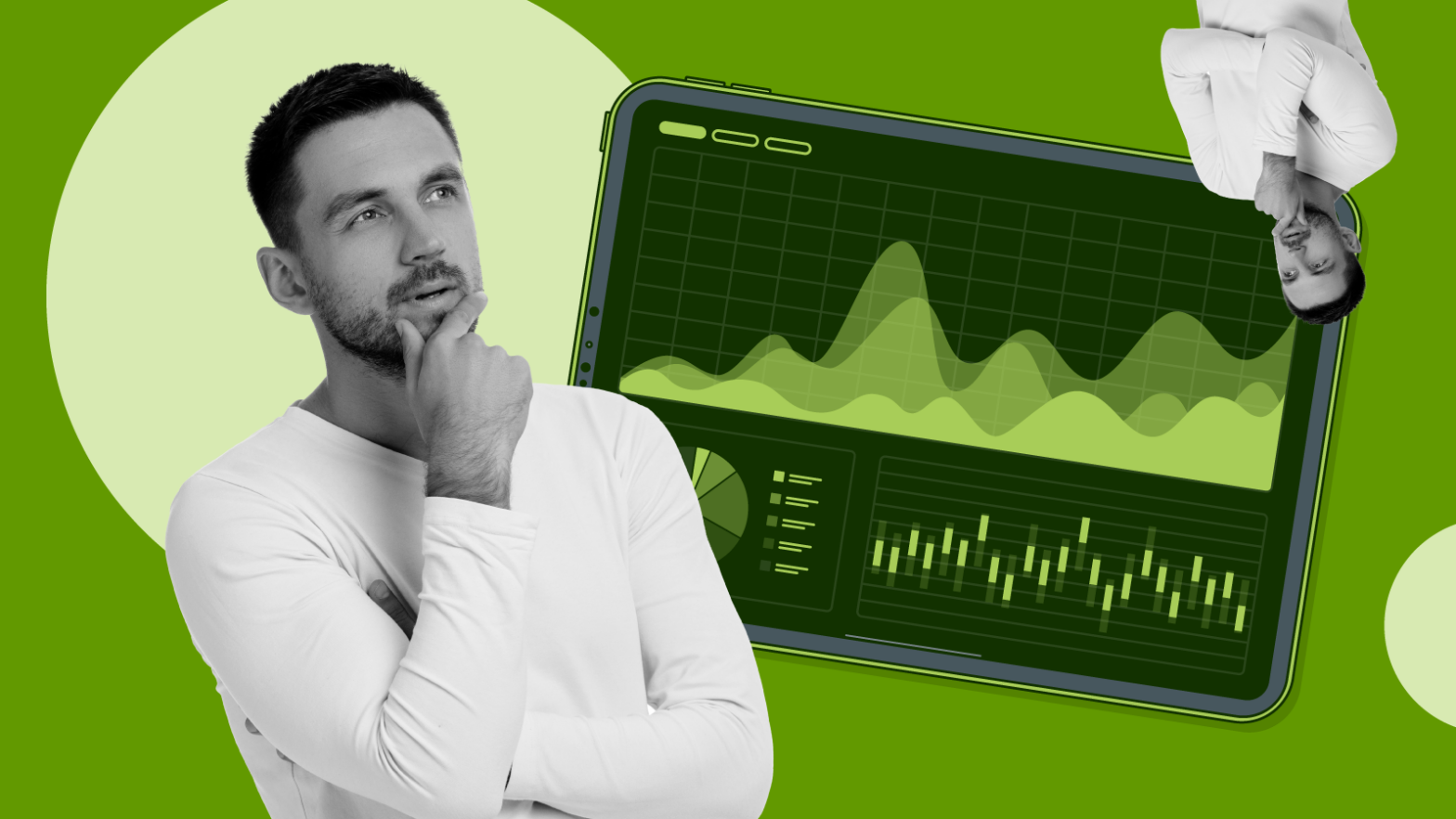
Organizations leveraging big data and analytics in HR operations are projected to see a 15% increase in productivity by 2024. In today’s data-driven business landscape, HR departments are evolving from administrative functions to strategic partners driving organizational success through analytics.
HR data analytics represents the intersection of human resources management and data science. At its core, HR data analytics (also known as people analytics or workforce analytics) is the systematic process of collecting, analyzing, and interpreting workforce-related data to make evidence-based decisions that enhance organizational performance and employee experience.
HR data analytics transforms raw information into actionable insights that directly impact business outcomes. It encompasses everything from recruitment metrics and performance evaluations to employee engagement scores and turnover rates. The practice goes beyond simple reporting of numbers to identifying patterns, predicting trends, and recommending actions based on complex data analysis.
What makes HR analytics powerful is its ability to connect people decisions to business results, showing how workforce strategies directly contribute to organizational goals like revenue growth, cost savings, and risk management.
- The importance of HR data analytics
- Core HR data analytics metrics
- Types of HR data analytics
- Step-by-step guide to implementing HR analytics
- Ethical considerations in HR data analytics
- Overcoming challenges in HR data analytics
- Case studies: Success stories in HR data analytics
- Future trends in HR data analytics
- 결론
- FAQs about HR data analytics
The importance of HR data analytics
In today’s competitive business environment, HR data analytics provides the critical intelligence needed to optimize human capital investments and drive business performance. According to Deloitte (2023), AI-powered performance management leads to a 71% increase in employee engagement and a 50% surge in goal achievement rates.
HR analytics transforms traditional human resources functions by providing evidence-based insights rather than relying on intuition or past practices. By analyzing patterns in recruitment, performance, engagement, and retention data, organizations can identify both problems and opportunities that might otherwise remain hidden.
Data-driven decision making
The true power of HR analytics lies in its ability to facilitate data-driven decision making across all personnel aspects. For example, analytics can reveal which recruitment sources yield the highest-performing employees, helping organizations allocate hiring budgets more effectively.
Companies implementing predictive analytics for talent management have achieved a 12.9% improvement in employee performance, with 86% of managers stating that AI enhances their effectiveness (Visier, 2023). These measurable improvements extend to reduced turnover costs, optimized compensation structures, and more effective talent development programs.
Core HR data analytics metrics
Understanding how to effectively track HR metrics is fundamental to successful HR analytics implementation. These quantifiable measures provide insights into various aspects of workforce management, helping organizations make informed decisions about their human capital strategies.
Essential HR metrics and calculations
1. Turnover metrics:
- Regrettable Turnover Rate = (Number of high performers who left voluntarily ÷ Total high performers) × 100
- Cost Per Turnover = (Recruitment costs + Onboarding costs + Lost productivity) ÷ Number of replacements
- Industry Benchmark: Financial services average 18.6% annual turnover, while healthcare averages 19.2% (2024)
2. Recruitment effectiveness:
- Time-to-Fill = Days between job posting and acceptance
- Quality of Hire = (Job performance score + Ramp-up time + Cultural fit score + Engagement score) ÷ 4
- Industry Benchmark: Average time-to-fill across industries is 42 days, with technology positions taking 56 days on average
3. Employee engagement:
- eNPS (Employee Net Promoter Score) = % Promoters – % Detractors
- Engagement Rate = (Number of engaged employees ÷ Total employees) × 100
- Industry benchmark: Average engagement rate is 36% across industries; top-performing companies achieve 71%
4. Productivity and performance:
- Revenue Per Employee = Total revenue ÷ Total number of employees
- Performance Distribution = Percentage of employees in each performance rating category
- Industry Benchmark: The adoption of machine learning in assessment processes has resulted in 20-30% higher accuracy rates in performance reviews, as reported by McKinsey in 2023
5. Diversity and Inclusion:
- Representation Rate = (Number of employees in demographic group ÷ Total employees) × 100
- Promotion Equity = Promotion rate of demographic group ÷ Overall promotion rate
- Industry Benchmark: The technology industry average for women in leadership is 28.8%; top performers reach 35%
These metrics provide a foundation for HR analytics, but the most valuable insights often come from analyzing relationships between different metrics. For example, correlating engagement scores with turnover rates can reveal the financial impact of employee satisfaction on retention.
Types of HR data analytics
HR data analytics encompasses several distinct approaches, each serving different purposes and offering unique insights. Understanding these different types helps organizations determine which methodologies best address their specific workforce challenges and strategic objectives.
The evolution of big data in HR has transformed workforce management. With the ability to process massive volumes of structured and unstructured data, HR departments can now gain unprecedented insights into employee behaviors, preferences, and performance patterns. This represents a fundamental shift from intuition-based decision making to evidence-based strategic planning.
Descriptive analytics
Descriptive analytics forms the foundation of HR analytics, focusing on understanding what has already occurred within the organization. This approach summarizes historical HR data to identify trends and patterns in workforce metrics. For example, descriptive analytics might reveal that employee turnover increased by 15% in the marketing department during the third quarter compared to previous years. These insights provide essential context for deeper analysis.
Diagnostic analytics
Moving beyond what happened, diagnostic analytics explores why certain events or trends occurred. This type of analysis examines relationships between variables to identify causal factors. For instance, by analyzing exit interview data alongside performance metrics, workload distribution, and compensation data, HR can pinpoint specific factors contributing to turnover in different departments or employee segments.
Predictive analytics
Predictive analytics applies statistical models and machine learning algorithms to historical and current data to forecast future workforce trends and employee behaviors. Predictive models might identify which high-performing employees are at risk of leaving in the next six months or project future skills gaps based on business growth trajectories and retirement forecasts.
Organizations implementing predictive analytics for talent management have achieved a 12.9% improvement in employee performance, with 86% of managers stating that AI enhances their effectiveness (Visier, 2023).
Prescriptive analytics
At the most advanced level, prescriptive analytics not only predicts future outcomes but also recommends specific actions to achieve desired results. This approach leverages artificial intelligence and complex algorithms to suggest optimal solutions to HR challenges. For example, prescriptive analytics might recommend personalized retention strategies for at-risk employees, optimal team compositions for specific projects, or individualized development paths to maximize employee potential and engagement.
Step-by-step guide to implementing HR analytics
Implementing HR data analytics effectively requires a structured approach that builds from foundational elements to advanced applications. This systematic process ensures that analytics initiatives deliver meaningful insights that drive organizational success rather than creating “data for data’s sake.”
1. Define clear business objectives
Start by clearly defining the business problems you want to solve through analytics. Effective HR data initiatives begin with specific questions tied to organizational priorities:
- “Why is turnover increasing in our technical teams?”
- “What factors predict sales performance in our organization?”
- “How effective are our learning and development investments in improving productivity?”
Having clear objectives ensures your analytics efforts address real business needs rather than generating interesting but ultimately unused insights.
2. Assess data quality and availability
Next, conduct an inventory of your existing HR data sources, including:
- HRIS systems
- Applicant tracking systems
- Performance management platforms
- Learning management systems
- Engagement surveys
- Compensation systems
Identify gaps in your data infrastructure and develop strategies to improve data quality through standardized collection methods, regular audits, and data governance frameworks. Remember that analytics insights are only as good as the data they’re based on—garbage in, garbage out.
3. Develop appropriate metrics and KPIs
Translate your business questions into specific, measurable indicators that provide meaningful insight. For instance, if retention is your focus, look beyond simple turnover rates to examine:
- Regrettable turnover (high performers who leave voluntarily)
- Retention by performance level
- Time-to-fill for critical positions
- Cost of replacement for key roles
- First-year turnover rates
Your metrics should directly connect to the business outcomes you’re trying to influence.
4. Select the right analytical techniques and tools
Different business questions require different analytical approaches:
- Descriptive analytics for understanding historical patterns
- Diagnostic analytics for identifying cause-and-effect relationships
- Predictive or prescriptive approaches for forward-looking insights
Choose tools that match your organization’s analytical maturity and technical capabilities, starting with simpler solutions if your analytics program is just beginning.
5. Transform insights into action
This crucial step bridges the gap between interesting information and meaningful organizational impact:
- Present findings in clear, compelling ways that resonate with different stakeholders
- Connect analytical insights directly to business outcomes when making recommendations
- Frame findings in terms of impact on organizational priorities like productivity, innovation, or financial performance
- Implement a process for ongoing monitoring and refinement of your analytics initiatives
- Establish feedback loops that measure the impact of decisions made using analytics insights
Remember that the most sophisticated analytics capability has limited value if decision-makers don’t trust or understand the insights it generates. Fostering a culture that values evidence over intuition in HR decision-making is often the greatest challenge in analytics implementation.
Ethical considerations in HR data analytics
As HR analytics capabilities advance, ethical considerations become increasingly important. Organizations must balance the power of data-driven insights with responsible practices that protect employee privacy and ensure fairness.
Data privacy and consent
Organizations must ensure they collect, store, and analyze employee data with proper consent and transparency. This includes being clear about what data is collected, how it’s used, and who has access to it.
Google emphasizes human input in data-driven decision-making, recognizing that analytics should complement rather than replace human judgment. Some organizations involve employees in designing performance evaluation systems to ensure transparency and build trust. Companies like Uber learned hard lessons after tracking employee movements without proper consent, highlighting the importance of establishing clear policies for data collection and handling.
Algorithmic bias and fairness
HR analytics tools can perpetuate or amplify existing biases if not carefully designed and monitored. Amazon discovered this challenge firsthand in 2015 when their data-based employee performance evaluation system was criticized for being unfairly harsh and lacking transparency.
After Amazon’s experience with biased algorithmic hiring processes, many organizations now conduct regular audits of their algorithms to identify and correct potential biases. Leading companies implement strict ethical guidelines and perform regular algorithm testing to ensure fair outcomes across different demographic groups.
Transparency and explainability
Employees should understand how analytics affect decisions about their careers and performance. Leading companies are adopting a “fair exchange of value” approach in people analytics, ensuring that data collection benefits both the organization and employees.
Some organizations now provide employees with access to their own data and insights about how analytics influence decisions regarding their careers. Progressive companies make algorithmic decision-making processes transparent, allowing employees to understand how conclusions are reached.
Balancing analytics with human judgment
Organizations must determine when to rely on data versus human expertise. Companies like Google have implemented human-centric approaches to HR analytics where data supports but doesn’t replace human decision-making.
Some organizations establish clear guidelines about which decisions can be fully automated versus which require human review. Leading companies provide training for managers on how to interpret and apply analytics insights alongside their professional judgment appropriately.
Data security and protection
Organizations must protect sensitive employee information from breaches and unauthorized access. Progressive companies implement comprehensive data governance frameworks that include regular security audits and strict access controls.
Some organizations establish dedicated ethics committees to oversee HR analytics practices and ensure compliance with both legal requirements and ethical standards. Leading firms conduct regular training on ethical data usage and implement robust security protocols to prevent breaches.
Studies suggest that up to 81% of people analytics projects face ethics and privacy concerns. By addressing these ethical considerations with thoughtful policies and practices, companies can harness the power of analytics while maintaining employee trust and organizational integrity.
Overcoming challenges in HR data analytics
Implementing HR data analytics successfully requires navigating several significant challenges that can derail even well-intentioned initiatives. Understanding these obstacles and developing strategies to address them is crucial for organizations looking to harness the full potential of workforce analytics.
Data integration complexity
Organizations typically store employee data across multiple systems, creating siloed information that’s difficult to consolidate. Successful organizations implement standardized data collection frameworks and governance policies to ensure consistency across sources.
One retail company overcame this challenge by implementing structured interview software that standardized candidate evaluation data, enabling more meaningful analysis of hiring effectiveness across diverse regional locations.
HR analytics skills gap
Traditional HR professionals may lack the statistical knowledge and technical skills required for sophisticated data analysis. Despite the growing importance of analytics, 60% of HR professionals struggled to prove the ROI of their initiatives in 2024, often due to budget constraints and difficulties in quantifying intangible gains.
Progressive organizations address this gap through targeted training programs and strategic hiring. Many HR professionals now pursue analytics certifications to develop these critical skills. Programs like Cornell’s HR Analytics certification or AIHR’s People Analytics course provide structured paths to building these competencies.
Data misinterpretation risks
Data misinterpretation can undermine analytics initiatives when HR professionals lack the statistical background to properly analyze workforce data. This challenge often manifests in correlation-causation confusion or selection bias in analysis.
For instance, one organization mistakenly attributed increased performance to a new training program, overlooking the fact that only high performers had been selected for participation. Establishing clear interpretation frameworks and involving statistical experts in the analysis process can help mitigate these risks.
Technology infrastructure limitations
Legacy HR systems may not support advanced analytics capabilities, while data security concerns complicate access to sensitive employee information. Cloud-based solutions have emerged as an effective response, offering scalable computing power without massive infrastructure investments.
Organizations like Google and Microsoft use their own cloud platforms to analyze workforce data securely while maintaining compliance with privacy regulations.
Change management challenges
Transitioning from intuition-based to data-driven HR decision-making represents a significant cultural shift. Resistance often stems from skepticism about data quality or fear that analytics will replace human judgment.
Successful implementations typically begin with focused projects addressing specific business problems rather than attempting comprehensive transformation. A healthcare organization demonstrated this approach by initially using analytics to analyze turnover patterns in nursing staff, generating immediate value that built credibility for broader analytics initiatives.
Case studies: Success stories in HR data analytics
Real-world examples of HR analytics implementation provide valuable insights into best practices and potential benefits. These success stories demonstrate how organizations across different industries have leveraged data to transform their HR functions and drive business outcomes.
IBM: Predictive retention analytics
IBM revolutionized its retention strategy through sophisticated predictive modeling. The company’s HR data analyst team developed an algorithm that could predict employee flight risk with 95% accuracy. By analyzing factors like compensation, job role, time since last promotion, manager effectiveness scores, and commute distance, IBM identified employees at risk of leaving before they began actively searching for new positions.
This proactive approach allowed managers to intervene with customized retention strategies, reducing voluntary turnover by 25% and saving an estimated $300 million in replacement costs and lost productivity.
Unilever: Data-driven recruitment
Unilever transformed its recruitment process through data-driven decision making. Facing challenges with traditional resume screening and interview methods, Unilever implemented AI-powered assessments that evaluated candidates on real-world business challenges, cognitive abilities, and behavioral traits.
The company used natural language processing to analyze interview responses and gamified assessments to measure problem-solving capabilities. The results were remarkable: a 90% reduction in time-to-hire, 16% increase in diversity of new hires, and a significant improvement in year-one performance ratings of new employees compared to traditional hiring methods.
Starbucks: Analytics informed benefits
Starbucks provides a compelling example of using analytics to enhance employee engagement and retention. Their analytics team discovered that healthcare benefits, while valuable to most employees, weren’t addressing the specific needs of their baristas, many of whom were college students.
By analyzing demographic data, engagement survey responses, and exit interviews, Starbucks developed their College Achievement Plan, offering full tuition coverage for online bachelor’s degrees. This data-informed benefit resulted in 50% higher retention rates among program participants and improved store performance metrics.
Google: Project Oxygen
Google’s Project Oxygen demonstrates how people analytics initiatives can challenge conventional wisdom. When Google’s HR analytics team examined performance data across the organization, they discovered that technical expertise, long considered the most important attribute for managers—ranked last among eight critical leadership qualities.
Instead, qualities like being a good coach, communicating clearly, and showing interest in team members’ success were the strongest predictors of high-performing teams. This insight fundamentally reshaped Google’s management development programs and evaluation criteria, resulting in significant improvements in team performance and manager ratings.
Healthcare provider: Predictive burnout prevention
A healthcare provider tackled burnout among clinical staff using predictive analytics. Their HR data analysts integrated electronic medical record usage patterns, shift scheduling data, and employee survey responses to identify early warning signs of burnout.
The predictive model allowed for personalized interventions, like schedule adjustments, mentoring opportunities, or wellbeing resources, before burnout symptoms significantly impacted performance or led to turnover. Within 18 months, the organization reduced turnover among nursing staff by 18% and improved patient satisfaction scores in departments where the model was implemented.
These case studies illustrate how organizations across industries are using HR analytics to transform traditional HR functions into strategic business drivers. Whether predicting turnover, enhancing recruitment, improving engagement, or challenging conventional wisdom, data-driven approaches deliver measurable improvements in workforce outcomes and business performance.
Future trends in HR data analytics
The landscape of HR analytics is rapidly evolving as technological advancements and shifting workforce dynamics create new possibilities for data-driven people management. Understanding emerging trends helps organizations stay ahead of the curve and leverage analytics strategically.
Advanced predictive workforce analytics
Organizations are increasingly using sophisticated algorithms to forecast future workforce scenarios, enabling proactive rather than reactive talent management strategies. Companies like Cisco are pioneering this approach by integrating internal HR data with external market information to predict skill gaps months before they materialize.
Automation and AI integration in HR processes are set to increase by 40% by 2025, halving administrative time and enabling HR professionals to focus on strategic initiatives.
AI and Machine Learning integration
Machine learning algorithms can now process millions of data points to identify subtle patterns in employee behaviors and outcomes that would be impossible to detect through traditional analysis. Natural language processing can analyze unstructured data from performance reviews, exit interviews, and engagement surveys to extract sentiment and predict outcomes.
The adoption of machine learning in assessment processes has resulted in 20-30% higher accuracy rates in performance reviews, as reported by McKinsey in 2023, while AI-powered performance management leads to a 71% increase in employee engagement and a 50% surge in goal achievement rates, according to Deloitte (2023).
Well-being analytics
Organizations are developing increasingly sophisticated methods to measure and support employee wellness across physical, mental, financial, and social dimensions. HR analytics approaches now incorporate information from multiple sources—including health apps, benefit utilization patterns, work hours, collaboration tools, and sentiment analysis—to create holistic well-being profiles.
One technology firm used analytics to identify that employees working more than 55 hours weekly showed significant productivity declines after eight weeks. This insight led to automated alerts when employees approached this threshold, prompting manager check-ins and additional support resources.
Skills mapping and development analytics
The accelerating pace of skill obsolescence has organizations shifting from traditional role-based talent management to skills-based approaches. Analytics platforms now map skills across the organization, identify emerging skill gaps, and recommend development pathways for employees.
These capabilities support more fluid internal talent marketplaces where employees can be deployed based on skills rather than job titles, increasing organizational agility and employee growth opportunities.
Enhanced DEI analytics
Organizations now use analytics to examine promotion rates, compensation patterns, performance evaluation consistency, and other indicators across demographic groups. These insights enable targeted interventions to address systemic barriers to inclusion and advancement.
The future of DEI analytics will increasingly incorporate external benchmarking and predictive capabilities to set meaningful goals and measure progress toward truly inclusive workplaces.
결론
HR data analytics has evolved from a specialized niche into an essential business function that drives strategic decision-making across organizations. The power of analytics lies not in the technology itself but in its application to solve real business challenges through better workforce management.
The journey toward data-driven HR requires thoughtful investment in infrastructure, skills development, and ethical frameworks. Organizations that successfully implement analytics programs establish clear connections between people metrics and business outcomes, ensuring that analytical insights translate into meaningful action.
Quality data serves as the foundation for effective analytics. Without this foundation, even the most sophisticated analytical techniques will yield flawed conclusions and erode trust in the analytics function. The most successful organizations treat data quality as an ongoing commitment rather than a one-time initiative.
Looking ahead, HR analytics will continue to evolve with advances in artificial intelligence, machine learning, and data visualization. Organizations that develop their analytical capabilities now position themselves to leverage these emerging technologies and maintain competitive advantage in increasingly dynamic talent markets.
As you begin or continue your HR analytics journey, remember that success comes not from analytical sophistication alone but from the ability to translate insights into action that improves employee experiences and organizational outcomes. By focusing on this ultimate goal, you’ll ensure that your analytics initiatives deliver lasting value in an increasingly data-driven world.
FAQs about HR data analytics
What is HR data analytics and how is it different from regular HR reporting?
HR data analytics involves collecting, analyzing, and interpreting workforce data to make strategic HR decisions and drive business outcomes. While traditional HR reporting typically focuses on descriptive statistics (what happened), HR data analytics delves deeper to understand patterns, relationships, and predictive insights. It’s the systematic application of statistical methods and data visualization techniques to HR data to enhance people-related decisions, moving beyond simple metrics tracking to identify causal relationships and predict future outcomes.
What are HR analytics’ primary benefits for organizations?
Key benefits include improved talent acquisition through data-driven candidate selection, enhanced employee retention by identifying flight risk factors, optimized workforce planning based on skills gaps analysis, and increased operational efficiency. Organizations implementing sophisticated analytics programs typically see 5-10% reductions in recruiting costs, 10-25% decreases in turnover, and measurable improvements in workforce productivity and engagement.
What types of data are typically used in HR analytics?
HR data analysis incorporates both structured and unstructured data from multiple sources, including demographic information, compensation data, performance metrics, learning and development records, engagement survey results, attendance patterns, and recruitment metrics. Advanced analytics programs may also incorporate external data sources like labor market statistics, competitor information, and economic indicators.
What skills are needed to implement HR analytics effectively?
Successful HR data analytics requires technical skills (statistical analysis, data visualization, database management) and business acumen (understanding organizational goals, translating data insights into actionable recommendations, communicating findings effectively). While not every HR professional needs advanced statistical knowledge, basic data literacy is becoming essential across HR functions.
How can organizations get started with HR analytics?
Begin with clearly defined business questions rather than jumping straight into data collection. Identify specific challenges where data could provide insights, such as “Why is turnover higher in certain departments?” Next, audit available data sources and quality. Start with simple analyses using existing tools before investing in specialized software. Focus initial projects on high-impact areas with readily available data, and gradually build complexity as you demonstrate value.
How does HR analytics support diversity, equity and inclusion initiatives?
HR analytics provides tools for measuring, tracking, and improving DEI outcomes by analyzing representation across different demographic groups, identifying potential bias in hiring and promotion processes, examining pay equity, and measuring inclusion through engagement survey results. Advanced analytics can also assess the impact of DEI initiatives and predict which interventions will be most effective.
What ethical considerations should guide HR analytics implementation?
Ethical HR analytics requires balancing data utility with employee privacy and fairness concerns. Organizations must ensure transparency about what data is collected and how it’s used, maintain appropriate data security, and comply with relevant regulations. Analytics models should be regularly tested for potential bias, particularly in applications like hiring or promotion recommendations. Successful organizations establish clear ethical guidelines and governance frameworks for their analytics programs.