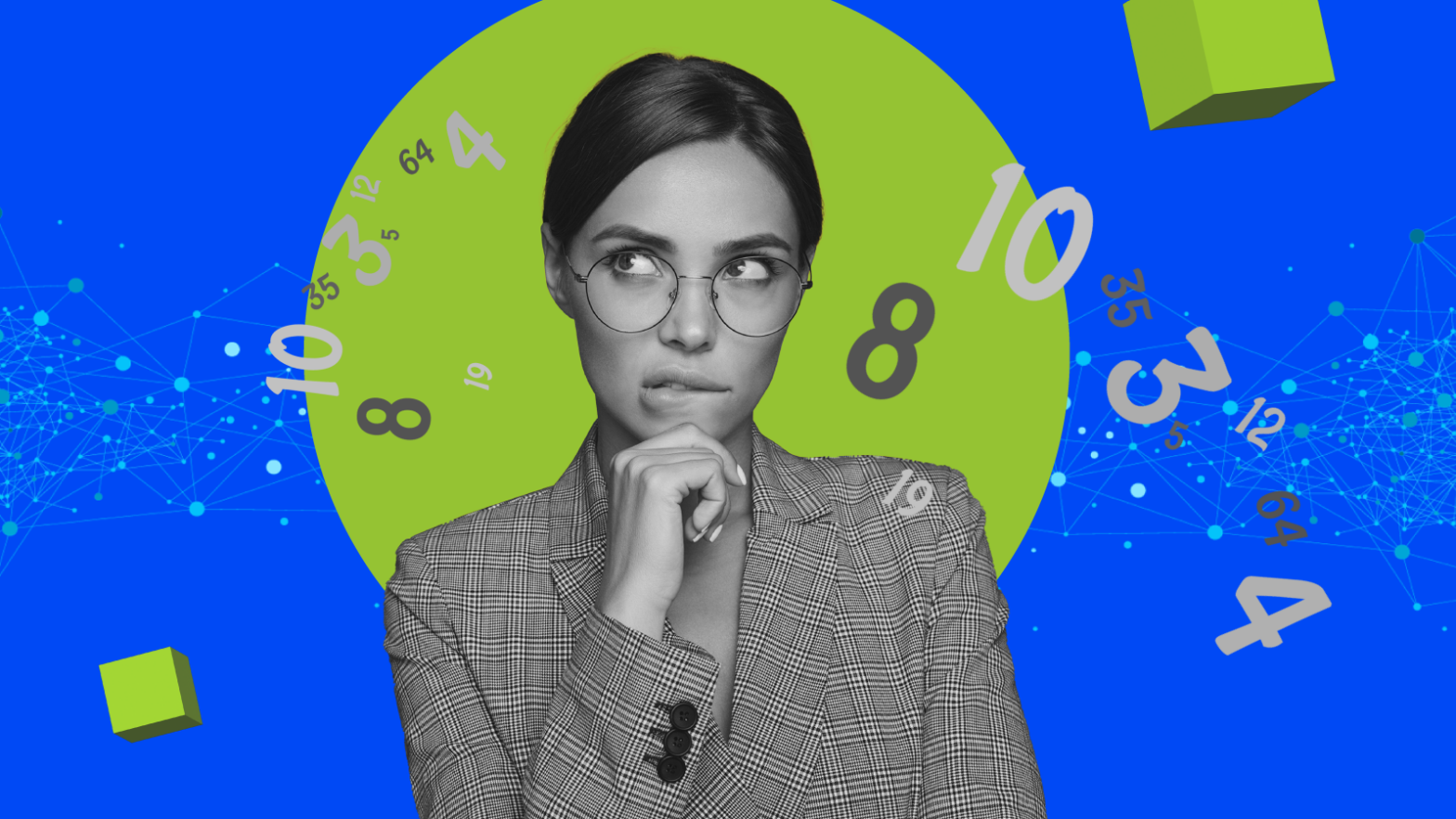
Talent analytics: A comprehensive guide
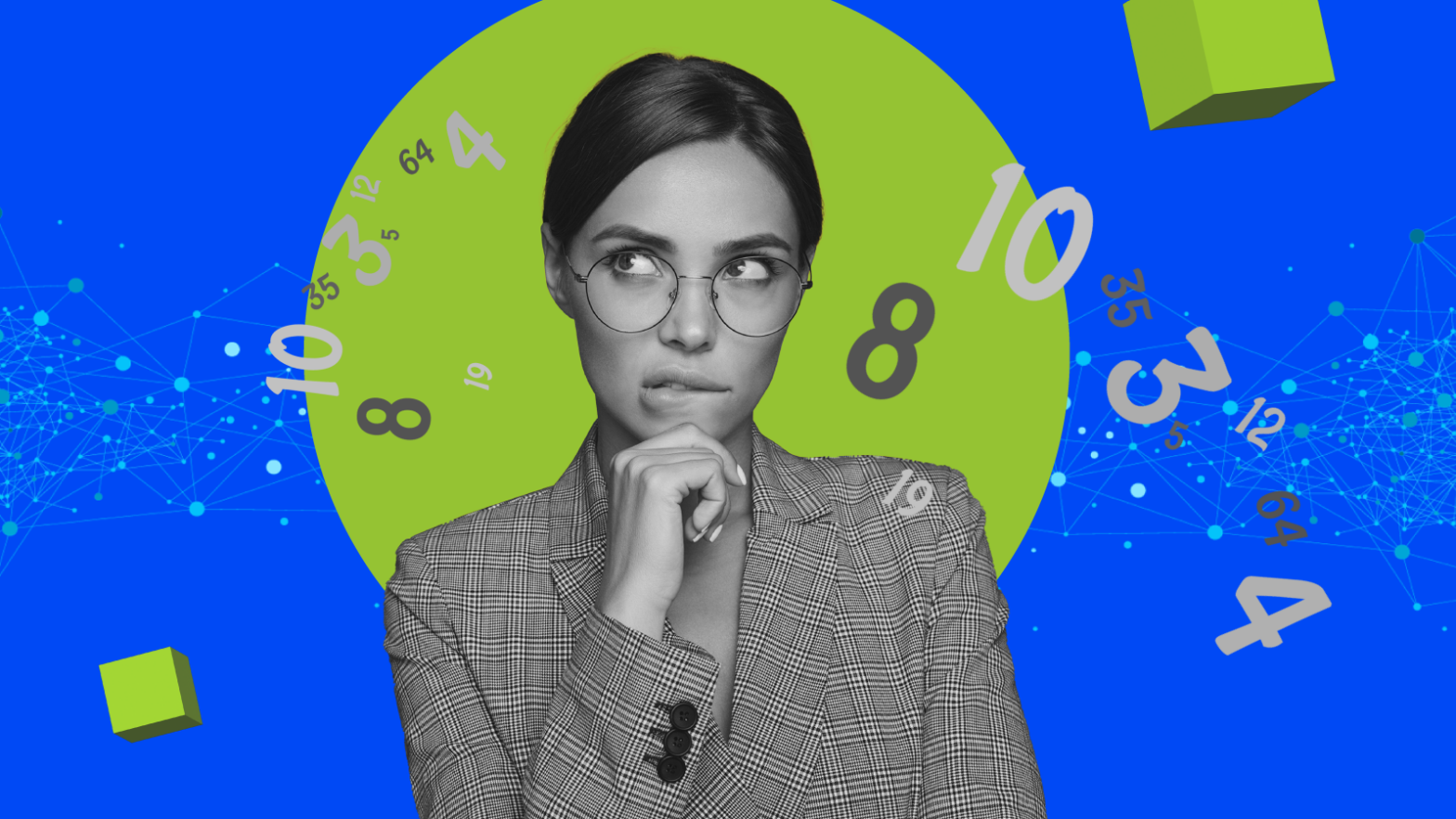
- Executive summary
- Understanding talent analytics: A comprehensive introduction
- The core components of talent analytics
- Industry-specific talent analytics
- The strategic benefits of utilizing talent analytics
- Implementing talent analytics in your organization
- Analyzing and interpreting talent data
- Case studies: Talent analytics in action
- Overcoming challenges in talent analytics
- Future trends in talent analytics
- Conclusion: The road ahead for talent analytics
- Getting started with talent analytics
Executive summary
Talent analytics represents the intersection of human resources management and data science, transforming how organizations understand and optimize their workforce. This guide explores how data-driven approaches improve talent acquisition, development, and retention while delivering measurable business outcomes. Key takeaways include:
- Organizations with mature talent analytics capabilities achieve 30% higher stock prices and 56% higher profit margins than competitors
- Effective talent analytics requires integration of descriptive, predictive, and prescriptive methodologies
- Industry leaders like Google, IBM, and Unilever demonstrate significant ROI through specific talent analytics implementations
- Emerging technologies including AI and machine learning are rapidly expanding analytical capabilities
- Implementation success depends on balancing technical capabilities with organizational adoption strategies
Understanding talent analytics: A comprehensive introduction
What is talent analytics?
Talent analytics represents the intersection of human resources management and data science. It involves collecting, analyzing, and interpreting workforce data to make evidence-based decisions about talent management. Unlike traditional HR approaches that rely on intuition, talent analytics harnesses data to drive strategic personnel decisions.
At its core, talent analytics transforms raw employee data into actionable insights that optimize workforce management. This approach covers everything from recruitment to performance management and succession planning. By leveraging statistical methods, companies can identify patterns that might otherwise remain hidden.
The strength of talent analytics lies in its ability to move HR from reactive to proactive decision-making. Organizations can anticipate challenges and opportunities, creating a more resilient workforce strategy rather than simply addressing problems after they arise.
The evolution and importance of talent analytics in modern workplaces
The journey of talent analytics began with basic HR metrics, tracking attendance and turnover rates. Today, it has evolved into sophisticated predictive modeling that forecasts employee performance and identifies flight risks before they materialize. This evolution reflects growing recognition that people are strategic assets critical to organizational success.
Digital transformation has accelerated this evolution, generating unprecedented amounts of workforce data. From applicant tracking systems to performance management platforms, modern HR technologies create rich data streams that offer valuable insights into workforce dynamics.
Organizations that fail to leverage talent analytics risk falling behind. The ability to make data-driven talent decisions has become a significant competitive advantage. Companies leading in talent analytics typically see 30% higher stock prices and 56% higher profit margins than their competitors.
How talent analytics powers HR decisions and business growth
Talent analytics bridges the gap between HR operations and business outcomes by quantifying the impact of people decisions on organizational performance. When implemented effectively, it enables HR to speak the language of business and demonstrate its strategic value.
For recruitment, talent analytics can identify which candidate sources yield the highest-performing employees, reducing time-to-hire while improving quality-of-hire. For retention, predictive models can flag employees at risk of leaving, allowing proactive intervention that saves both institutional knowledge and replacement costs.
Beyond tactical applications, talent analytics informs strategic workforce planning by providing insights into skill gaps and succession planning needs. This forward-looking perspective allows organizations to align their talent strategies with long-term business objectives, ensuring they have the right people in the right roles at the right time.
The core components of talent analytics
Unlocking the full potential of talent analytics requires understanding its fundamental components—the types of analytics methodologies, key metrics that drive decision-making, and the technological ecosystem that enables advanced workforce analysis.
Types of talent analytics: Descriptive, predictive, and prescriptive
Talent analytics operates across three distinct yet complementary approaches representing an evolution in analytical sophistication:
Descriptive analytics forms the foundation by answering “What happened?” This retrospective approach examines historical workforce data to identify patterns and trends. For example, descriptive analytics might reveal that turnover increased by 15% in engineering while remaining stable elsewhere. This historical perspective provides context for understanding current workforce dynamics.
Predictive analytics elevates talent reporting to forward-looking insights by addressing “What might happen next?” Using statistical models and machine learning, organizations can forecast future talent scenarios. A predictive model might determine that employees with specific profiles have a 70% probability of leaving within 18 months without intervention. This enables proactive workforce planning rather than simply cataloging past events.
Prescriptive analytics represents the most sophisticated tier, answering “What should we do about it?” This approach doesn’t just predict outcomes but recommends specific actions to achieve desired results. For instance, prescriptive analytics might suggest targeted compensation adjustments for high-flight-risk employees in critical roles, quantifying the expected impact on retention rates.
Key metrics in talent analytics: What to measure
Effective talent analytics hinges on tracking metrics that align with organizational priorities:
Recruitment metrics provide crucial insights into talent acquisition effectiveness. Time-to-fill indicates process efficiency, while cost-per-hire helps optimize recruitment spending. Quality-of-hire offers insights into whether recruitment efforts are bringing in the right talent. Source effectiveness tracking reveals which channels deliver the highest-quality candidates.
Retention and engagement metrics help organizations understand workforce stability. Employee turnover rate serves as a vital health indicator, while retention by department can spotlight problem areas. Engagement scores correlate strongly with productivity and innovation. Absence patterns may reveal underlying engagement issues before they manifest as turnover.
Performance metrics connect people data to business outcomes. Individual performance scores, when aggregated, reveal team effectiveness. Productivity measures link human capital investments to tangible outputs. Skills gap analysis identifies where training investments should be directed. High-performer profiles help organizations understand the attributes that drive exceptional results.
These metrics gain power when analyzed together rather than in isolation. For example, correlating engagement scores with performance metrics might reveal that highly engaged teams are 21% more productive—a finding that can justify specific culture investments.
Tools and technologies supporting talent analytics
The talent analytics ecosystem continues to evolve, with specialized tools enabling increasingly sophisticated analysis:
Core talent analytics platforms integrate data from multiple HR systems to create unified workforce views. Solutions like Visier and ChartHop consolidate fragmented employee data, enabling comprehensive analysis across the employee lifecycle. These platforms offer pre-built analytics models tailored to common workforce challenges.
Function-specific analytics tools address particular HR domains. Recruitment analytics tools like Lever and SmartRecruiters offer insights into talent acquisition funnels. Performance management systems such as 15Five and Lattice provide visibility into productivity drivers. Learning analytics platforms track skills development across the organization.
Advanced technology enablers are extending what’s possible in talent analytics. Machine learning algorithms power sophisticated flight risk models that predict employee departures with increasing accuracy. Natural language processing extracts insights from unstructured feedback data. Network analysis tools map collaboration patterns within organizations.
When selecting talent analytics tools, organizations should prioritize solutions that balance analytical capabilities with intuitive interfaces accessible to HR professionals who may not have data science backgrounds.
Industry-specific talent analytics
TECH INDUSTRY FOCUS
Technology companies should prioritize tracking employee turnover rate, as the tech sector typically experiences higher turnover than other industries. Performance metrics like revenue per employee and project completion efficiency directly correlate with operational effectiveness. Tech firms should also measure skills obsolescence rate to address rapidly evolving technical requirements, and track innovation contribution through metrics like patents filed and new product ideas implemented.
The strategic benefits of utilizing talent analytics
The value of talent analytics extends beyond collecting workforce data—it transforms how organizations approach their human capital strategy. Forward-thinking companies realize that data-driven HR practices deliver measurable competitive advantages across the employee lifecycle.
Enhancing recruitment and hiring efficiency
Talent analytics revolutionizes recruitment by enabling precision in candidate acquisition and selection. By analyzing historical hiring data, organizations can identify which candidate sources yield the highest-performing employees. One global technology firm discovered through analytics that candidates from specific universities excelled in technical roles but underperformed in client-facing positions.
The efficiency gains from analytics-driven recruitment are substantial. Companies implementing talent analytics in hiring report an average 17% reduction in time-to-fill positions and a 26% decrease in cost-per-hire. These improvements stem from more targeted job advertising, streamlined screening processes, and data-backed candidate evaluations.
Perhaps most importantly, talent analytics enables predictive quality-of-hire assessments. By correlating pre-hire assessments with post-hire performance data, organizations can continuously refine their selection criteria, creating a virtuous cycle where each hiring decision improves future hiring models.
Reducing employee turnover and retention challenges
Employee turnover represents one of the costliest challenges organizations face, with replacement costs ranging from 50-200% of an employee’s annual salary. Talent analytics provides tools to combat this challenge by identifying turnover patterns and predicting flight risks before resignations occur.
Through regression analysis of historical turnover data, organizations can identify specific factors correlating with employee departures. These predictive models often reveal surprising insights—one healthcare organization discovered that commute distance was a stronger predictor of turnover than compensation for certain roles. Armed with this knowledge, they adjusted their recruiting radius and implemented remote work options, reducing turnover by 23%.
The benefits extend to proactive retention interventions. By identifying high-risk employees, organizations can initiate personalized retention strategies before staff reach resignation decision points. Companies effectively deploying these predictive retention models report an average 20% reduction in voluntary turnover among high performers.
FINANCIAL SERVICES FOCUS
Financial institutions operate in a highly regulated environment where regulatory compliance performance metrics are critical. Tracking revenue generation per employee helps understand the effectiveness of revenue-generating staff in areas like investment banking and wealth management. Cross-selling efficiency measures how effectively employees can sell multiple products to existing customers—a key growth strategy. Client retention linked to relationship managers directly impacts long-term profitability in this relationship-driven industry.
Improving workforce productivity and performance
Talent analytics transforms performance management from an annual ritual to a data-driven, continuous improvement process. By analyzing performance patterns across teams, organizations can identify the specific management practices and workplace conditions that enable peak performance.
One retail organization discovered through talent analytics that stores with managers who conducted brief daily team huddles outperformed other locations by 12% in sales productivity. This insight, which emerged from correlating management practices with performance metrics, was subsequently implemented across all locations with significant positive impact.
Analytics also reveals the true impact of training investments. By tracking performance metrics before and after specific training interventions, organizations can calculate precise ROI on development programs. Companies that leverage talent analytics for training optimization report achieving the same performance improvements with 40% less training expenditure.
PayPal has demonstrated tangible financial benefits from their talent analytics initiatives. Their analysis showed that reducing employee turnover by just 1% would result in approximately $500,000 in annual savings through improved employee productivity and reduced training costs.
Supporting diversity and inclusion initiatives
Data-driven approaches to diversity and inclusion move organizations beyond good intentions to measurable progress. Talent analytics provides visibility needed to identify specific points where diversity challenges emerge—whether in sourcing, selection, promotion, or retention processes.
Philips exemplifies this approach, having used talent analytics to set concrete diversity goals after identifying specific representation gaps. By analyzing where diverse candidates were falling out of their recruitment funnel, they implemented targeted interventions that increased diversity while maintaining hiring quality standards.
The business impact extends beyond representation metrics. TechCorp’s implementation of blind resume reviews and data-informed outreach programs resulted in not just a 30% increase in representation of underrepresented groups but also a measurable 22% improvement in overall employee satisfaction scores. This illustrates how talent analytics connects diversity initiatives to broader organizational performance.
Implementing talent analytics in your organization
Moving from theoretical benefits to practical implementation requires a strategic approach that addresses organizational structure, goal alignment, and data management fundamentals. The journey to becoming a data-driven HR function doesn’t happen overnight—it requires careful planning and the right expertise.
Building a talent analytics team: Roles and responsibilities
Creating an effective talent analytics team demands thoughtful consideration of required skill sets and organizational structure. The ideal team combines technical data expertise with deep HR knowledge and business acumen.
The People Analytics Leader serves as the strategic architect, connecting analytics initiatives to business priorities. This role bridges the gap between technical analytics work and executive decision-making, requiring data literacy, HR expertise, and business strategy understanding. The analytics leader must translate complex findings into compelling narratives for C-suite leaders.
Data Scientists and Analysts form the technical backbone of the talent analytics team. Data scientists design predictive models forecasting workforce trends and attrition risks. Analysts conduct descriptive analyses, create dashboards, and perform ad-hoc investigations to answer specific business questions. Together, they transform raw workforce data into meaningful insights.
HR Business Partners serve as critical translators between the talent analytics team and frontline managers. They help identify business challenges that analytics can address, contextualize findings within specific business units, and facilitate implementation of data-driven interventions. Their understanding of day-to-day HR operations ensures analytics projects deliver practical value.
Data Engineers and IT Specialists provide the technical infrastructure enabling analytics work. They build data pipelines that integrate information from disparate HR systems, ensure data quality and security, and develop the architecture that allows for scalable analytics capabilities.
HEALTHCARE INDUSTRY FOCUS
Healthcare organizations should track patient satisfaction scores by department to understand how different teams affect patient experiences. Clinical staff productivity metrics like patients served per practitioner help optimize staffing while maintaining quality care standards. Tracking certification and continuing education completion is essential in healthcare’s highly regulated environment. Interdepartmental collaboration metrics can highlight opportunities to improve integrated care delivery, which is particularly important in healthcare settings.
Defining objectives and aligning with business goals
Successful talent analytics initiatives begin with clear alignment to strategic business objectives. Rather than analyzing data simply because it’s available, organizations should approach talent analytics with specific business questions in mind.
The most impactful programs directly connect people metrics to business outcomes. For example, rather than merely tracking training completion rates, organizations can analyze how specific learning initiatives impact productivity or customer satisfaction. This business-centric approach ensures talent analytics delivers tangible value beyond HR process improvements.
Prioritization becomes essential as organizations typically identify more potential analytics projects than they can reasonably execute. Effective frameworks assess potential initiatives based on business impact, feasibility, and strategic alignment. This prevents the talent analytics team from becoming overwhelmed by requests or focusing on technically interesting but strategically peripheral questions.
Establishing clear success metrics for analytics initiatives enables organizations to demonstrate ROI and build continued support for investments. These metrics might include direct financial impacts (like reduced recruitment costs), operational improvements (such as decreased time-to-hire), or workforce outcomes (including improved retention).
Organizations with strong learning cultures enabled by analytics experience 57% higher employee retention rates compared to those without such cultures, demonstrating the business impact of analytics-informed talent development strategies.
Collecting and managing relevant data
The foundation of effective talent analytics is high-quality, accessible data. Organizations must establish systematic approaches to data collection, integration, cleaning, and governance to enable reliable analyses.
Data mapping exercises help organizations understand what workforce information currently exists, where it resides, and what gaps need addressing. Many discover they have rich data on recruitment and compensation but limited visibility into skills, internal mobility, or detailed performance metrics. Identifying gaps early allows for strategic data collection planning.
Data integration challenges often present significant hurdles for talent analytics initiatives. HR information typically resides in multiple systems—applicant tracking systems, HRIS platforms, learning management systems, performance tools, and more. Creating a unified data architecture requires thoughtful planning and specialized data engineering expertise.
Data quality and governance deserve particular attention given the sensitive nature of workforce information. Establishing clear data standards, ownership, and maintenance procedures ensures analytics work builds on a trustworthy foundation. Organizations should develop explicit policies regarding data privacy, anonymization practices, and compliance with regulations like GDPR or CCPA.
Many organizations leverage specialized talent analytics platforms that combine data integration capabilities with purpose-built visualization and analysis tools. These platforms offer advantages in implementation speed and HR-specific functionality compared to generic business intelligence tools.
MANUFACTURING INDUSTRY FOCUS
Manufacturing firms should closely monitor production efficiency per employee to understand how individual and team performance affects operational outputs. Safety compliance and incident rates help identify risks before accidents occur in these physically demanding environments. Machine operator proficiency measures how quickly operators reach proficiency and maintain production standards. Multi-skilling index—the percentage of employees trained in multiple skills/stations—indicates workforce flexibility and resilience against absenteeism.
Analyzing and interpreting talent data
Once you’ve established your talent analytics foundation, the true value emerges through rigorous analysis, interpretation, and action. This critical phase transforms raw workforce data into strategic insights that drive organizational performance.
Statistical methods and analytical techniques
Talent analytics leverages a range of statistical methods to extract meaningful patterns from workforce data. Each technique serves different analytical purposes and answers specific types of talent questions.
Regression analysis stands as one of the most versatile tools, helping organizations understand relationships between variables and predict outcomes. For example, multiple regression can identify which factors most strongly correlate with employee performance, distinguishing between the impacts of experience, education, and training completion. This enables HR leaders to focus development investments on variables with the greatest performance impact.
Cluster analysis groups employees with similar characteristics, revealing natural segments within your workforce. This proves particularly valuable for personalization strategies—organizations can identify distinct employee segments with different engagement drivers, enabling tailored retention approaches. One retail organization discovered through cluster analysis that their millennial employees valued growth opportunities most highly, while mid-career professionals prioritized work-life balance.
Survival analysis, borrowed from epidemiology, helps predict employee tenure and identify attrition risk factors over time. Unlike simple turnover metrics, it considers time dimensions and changing risk patterns. This technique revealed to one organization that their highest attrition risk occurred at specific tenure milestones (18 months and 4 years), allowing for targeted retention interventions at these critical periods.
Advanced talent analytics programs increasingly incorporate machine learning algorithms that can process larger datasets and identify more complex patterns than traditional statistical methods. These algorithms excel at predictive tasks like identifying flight risks or forecasting future skill needs.
Transforming insights into actionable strategies
The greatest analytical insights provide little value unless translated into concrete actions. Effective talent analytics programs build systematic pathways from data to decisions.
The insight-to-action framework begins with clear problem definition before analysis starts. Organizations should articulate specific business questions they hope to answer—whether addressing retention challenges, optimizing recruitment channels, or enhancing performance management. This clarity ensures analyses produce relevant findings rather than interesting but ultimately unused insights.
Cross-functional insight teams enhance the translation process. By including representatives from HR, operations, finance, and relevant business units in interpreting findings, organizations ensure broader perspectives on potential actions and increase stakeholder buy-in. This collaborative approach bridges the gap between technical analysis and practical implementation.
Action planning workshops structure the transition from insight to initiative. These facilitated sessions bring together key decision-makers to review findings, brainstorm potential responses, evaluate implementation feasibility, and prioritize actions. Without this structured approach, organizations often face “analysis paralysis” where insights fail to generate meaningful change.
Companies using analytics to provide personalized career advancement and skills development opportunities save an estimated $8,053 per employee annually through increased productivity, decreased employee churn, and lower healthcare costs.
Monitoring and continuously improving performance
Talent analytics delivers the greatest value when approached as an ongoing performance improvement cycle rather than a series of discrete projects.
Performance dashboards provide real-time visibility into key talent metrics, enabling proactive management rather than reactive responses. Effective dashboards balance comprehensiveness with clarity, highlighting critical indicators while allowing drill-down into detailed metrics when needed. The best dashboards customize views for different stakeholders—executives might see organization-wide retention trends while line managers access team-specific engagement metrics.
Regular insight reviews institutionalize data-driven talent management. Monthly or quarterly sessions dedicated to reviewing talent analytics findings ensure workforce insights remain central to business planning. These reviews should include not just HR leaders but also operational executives who can connect talent patterns to business outcomes.
Continuous feedback loops refine both analytics approaches and resulting actions. The most mature organizations systematically evaluate which analyses yield the most valuable insights and which actions most effectively address identified challenges. This learning orientation prevents talent analytics from becoming a static reporting exercise.
Case studies: Talent analytics in action
Google’s project Oxygen
Google used advanced data analysis to challenge assumptions about effective management. By analyzing performance reviews, employee surveys, and other data sources, they identified eight key behaviors that defined successful managers—with technical expertise ranking surprisingly low on the list. This data-driven approach led to a complete redesign of Google’s management development program, resulting in a 75% improvement in manager quality scores. The project demonstrated how talent analytics can transform subjective aspects of workplace dynamics into measurable dimensions.
IBM’s retention prediction model
IBM developed sophisticated algorithms analyzing over 70 variables to identify employees at risk of leaving. The system predicts attrition risk with over 95% accuracy, allowing managers to intervene before high-value employees depart. The model discovered counterintuitive insights—for instance, employees who hadn’t changed roles recently were more likely to leave than those who had, contradicting conventional wisdom about job stability. This initiative reportedly saved IBM over $300 million in replacement costs and retained crucial institutional knowledge.
Unilever’s recruitment transformation
Unilever replaced traditional campus recruitment with a digital-first approach incorporating gamified assessments, video interviews, and AI-powered analysis. The system evaluates candidates on hard and soft skills while minimizing unconscious bias. The results proved transformative: application numbers increased by 300%, time-to-hire decreased from four months to four weeks, and cost-per-hire fell by 90%. Most importantly, diversity of new hires increased significantly while retention rates improved, demonstrating that data-driven recruitment can enhance both efficiency and quality.
Overcoming challenges in talent analytics
While talent analytics offers tremendous potential for transforming HR and driving business outcomes, organizations frequently encounter significant hurdles during implementation. Understanding these challenges and developing strategies to overcome them is crucial for realizing the full value of talent analytics investments.
Data privacy and ethical considerations
The expansion of talent analytics capabilities has created unprecedented ethical dilemmas. With the capacity to collect and analyze vast amounts of employee data comes significant responsibility to use these capabilities ethically.
Privacy regulations like GDPR in Europe, CCPA in California, and similar frameworks worldwide have established strict guidelines for handling personal data. These regulations impact talent analytics by requiring explicit consent for data collection, ensuring data minimization, and providing employees rights to access and delete their information. Organizations must develop clear data governance policies that address these requirements while enabling meaningful analysis.
Beyond legal compliance, ethical considerations should guide talent analytics practices. The “mosaic effect”—where combining seemingly innocuous datasets reveals sensitive information about individuals—represents a particular concern. Organizations should establish ethical review processes for talent analytics initiatives that consider potential unintended consequences.
Transparency builds trust in talent analytics programs. Employees should understand what data is being collected, how it’s being used, and what safeguards are in place. Organizations that communicate openly about their practices typically encounter less resistance than those that implement analytics without clear communication.
Handling data silos and integration issues
The fragmented nature of HR technology landscapes presents a significant challenge for comprehensive talent analytics. Most organizations manage workforce data across multiple systems—recruitment platforms, HRIS cores, performance management tools, and learning systems—creating data silos that impede holistic analysis.
Data integration strategies should prioritize connecting these disparate systems to create unified talent profiles. API-based integration approaches offer flexibility by allowing specialized systems to exchange information without requiring complete system replacement. For organizations with mature data capabilities, data lakes or warehouses can consolidate information while maintaining the specialized functionalities of individual HR systems.
Data standardization addresses the “apples to oranges” comparison challenge that frequently undermines talent analytics initiatives. When different business units use inconsistent terminology, metrics, or collection methods, meaningful cross-organization analysis becomes impossible. Establishing enterprise-wide data standards creates the foundation for reliable talent analytics.
Master data management for core employee information ensures consistency across systems. Organizations should establish authoritative sources for critical data elements with governance processes ensuring this information remains aligned across all connected systems. This “single source of truth” approach prevents conflicting results that undermine confidence in findings.
Change management and organizational buy-in
Even the most sophisticated talent analytics capabilities deliver little value without organizational adoption. Cultural resistance to data-driven HR practices often represents a more significant barrier than technical challenges.
Executive sponsorship provides critical momentum for talent analytics initiatives. When senior leaders actively champion data-driven decision-making, demonstrate personal use of analytics, and allocate appropriate resources, they signal the strategic importance of talent analytics. Organizations should identify and cultivate executive champions who understand both the potential business impact and required organizational changes.
Data literacy development helps close the capability gap that often impedes talent analytics adoption. Many HR professionals and line managers lack experience working with data and may feel intimidated by analytical approaches. Progressive organizations are investing in talent analytics training programs that build basic analytical skills and develop critical thinking about data interpretation.
Early wins build momentum and credibility for talent analytics programs. Rather than beginning with complex, enterprise-wide initiatives, organizations should identify focused use cases that address clear business pain points and can demonstrate value quickly. For example, analyzing recruitment channel effectiveness to optimize sourcing investments typically delivers faster ROI than comprehensive workforce planning models.
74% of HR professionals report improved job performance in their organizations after implementing analytics-driven upskilling programs, showing how talent analytics can deliver measurable performance improvements.
Future trends in talent analytics
The landscape of talent analytics is evolving rapidly, driven by technological advancements and shifting workforce dynamics. As we look toward the future, several transformative trends are emerging that will reshape how organizations understand and manage their talent.
Artificial intelligence and machine learning in talent analytics
Artificial intelligence and machine learning represent the most transformative forces in the evolution of talent analytics. These technologies are moving talent analytics from descriptive and diagnostic approaches toward increasingly sophisticated predictive and prescriptive capabilities.
Natural language processing (NLP) is revolutionizing how organizations extract insights from unstructured data. Advanced algorithms can analyze resumes, performance reviews, employee feedback, and communication patterns to identify subtle indicators of engagement or flight risk. Some organizations implement sentiment analysis of internal communications to create real-time employee engagement pulses that complement traditional surveys.
Automated candidate screening represents one of the most mature AI applications. Organizations implementing AI-powered screening report 85% reductions in time-to-shortlist while increasing quality-of-hire metrics. The most advanced systems move beyond keyword matching to understand conceptual skills, assess culture fit probabilities, and predict performance potential based on subtle resume indicators validated against historical data.
Predictive modeling for employee attrition has reached remarkable levels of sophistication. Companies like IBM utilize machine learning algorithms analyzing over 70 variables to predict flight risk with 95% accuracy up to 12 months before likely resignation. These models identify not just who might leave but why, enabling targeted retention interventions.
Career pathing algorithms use machine learning to identify personalized development journeys. By analyzing thousands of career progression patterns, these systems can recommend optimal next roles, required skill development, and likely timeframes for advancement based on an individual’s profile and aspirations. Leading organizations use these tools to create “talent marketplaces” that match internal opportunities with employee capabilities.
However, AI implementation brings significant challenges. Algorithmic bias remains a critical concern, with AI systems potentially perpetuating historical biases present in training data. Leading organizations implement rigorous bias detection and mitigation strategies, including diverse training data requirements and regular algorithmic audits.
The impact of emerging technologies on talent insights
Beyond AI and machine learning, several emerging technologies are expanding the possibilities for talent analytics by creating new data sources and analytical approaches.
Advanced sensing technologies create unprecedented visibility into workplace interactions. Organizational network analysis (ONA) tools map collaboration patterns, identifying informal influence networks, collaboration bottlenecks, and innovation clusters that traditional reporting structures miss. Smart workplace technologies track physical interaction patterns, showing how office layouts affect collaboration frequency and quality.
Blockchain technology is beginning to transform credential verification and skill validation. Blockchain-based credential systems create tamper-proof records of education, certifications, and work histories that eliminate verification delays in hiring processes. Some organizations are exploring blockchain-based skill validation where demonstrated capabilities are recorded as verifiable “tokens” that follow individuals throughout their careers.
Virtual and augmented reality technologies enable new approaches to skill assessment and development tracking. VR-based simulations can assess complex skills in realistic scenarios, providing more accurate predictive data than traditional interviews. Some organizations use AR systems that overlay performance data and learning needs in real-world work contexts, creating continuous feedback loops that generate rich data on skill development.
The growing API ecosystem is transforming talent data integration possibilities. Rather than monolithic HR systems, many organizations adopt best-of-breed solutions connected through robust APIs. This approach enables talent analytics to incorporate data from multiple specialized systems into unified talent data lakes, providing more comprehensive insights than possible when data remained siloed.
Conclusion: The road ahead for talent analytics
As we look toward the future, talent analytics stands at a pivotal intersection of human resource management, data science, and business strategy. Organizations that thrive will be those that implement sophisticated analytical tools while developing the organizational capabilities to translate workforce insights into strategic advantage.
Talent analytics has evolved from simple HR reporting to a sophisticated discipline driving strategic decision-making across the employee lifecycle. The fundamental shift has been from backward-looking metrics to forward-looking predictive and prescriptive insights enabling proactive talent management. This evolution mirrors the broader transformation of HR from an administrative function to a strategic business partner.
The strategic benefits extend far beyond operational HR improvements. Organizations leveraging advanced talent analytics report significant competitive advantages through enhanced recruitment efficiency, reduced turnover costs, improved workforce productivity, and more diverse talent pools. These outcomes translate directly to bottom-line results.
To maintain competitive advantage in talent analytics, organizations should focus on aligning initiatives with business strategy, investing in data quality, building analytical literacy across the organization, developing ethical frameworks for data use, and fostering experimental mindsets that embrace continuous learning. This balanced approach ensures talent analytics delivers sustained business value.
The future belongs to organizations that view talent analytics not as a technical implementation but as a fundamental capability transforming how they understand and optimize their workforce. By connecting human insight with data science, talent analytics bridges the historical divide between intuitive people management and evidence-based decision-making.
As we navigate this evolving landscape, successful organizations will maintain a human-centered perspective, using technology to augment rather than replace human judgment. Talent analytics at its best doesn’t dehumanize workforce decisions—it makes them more humane by replacing biases with evidence and assumptions with insights.
Getting started with talent analytics
To begin implementing talent analytics in your organization, focus on these key actions:
- Define specific business problems to solve with analytics rather than starting with available data
- Conduct a data inventory to understand what workforce information exists and where quality gaps might be
- Start with focused, high-impact projects that can demonstrate value quickly
- Build necessary skills through targeted training or strategic partnerships
- Establish clear data governance and ethical guidelines from the outset
Companies that use analytics to allow employees to influence their learning paths see employees nearly eight times more likely to advance within the organization and over five times more likely to be high performers, showing the power of analytics-driven personalization.
The journey to mature talent analytics capabilities typically unfolds over years rather than months. However, by starting with clear business objectives and building capabilities incrementally, organizations can begin generating valuable workforce insights that drive measurable business impact today.